Understanding CF Data: A Comprehensive Guide
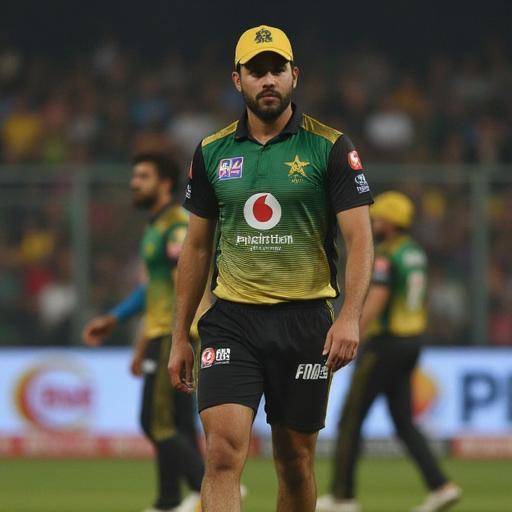
Unlocking the Power of CF Data: A Comprehensive Guide
Are you looking to harness the insights hidden within CF data? This in-depth guide will equip you with the knowledge and understanding needed to navigate this complex landscape and extract meaningful information. From the basics to advanced applications, we’ll explore the various facets of CF data and its potential applications.
What is CF Data, Anyway?
CF data, or Collaborative Filtering data, refers to a sophisticated technique used in recommendation systems. Instead of relying solely on explicit user ratings or reviews, CF data analyzes patterns in user behavior to predict what items a user might enjoy. Imagine Netflix suggesting movies you’ve never seen but might love based on your past viewing habits – that’s the magic of CF data in action.
Why Should You Care About CF Data?
In today’s data-driven world, CF data offers immense potential for enhancing user experiences, improving business strategies, and driving revenue growth. Businesses leveraging CF data can create highly personalized recommendations that significantly increase engagement and satisfaction. It allows for the prediction of customer preferences, leading to smarter product placement, more targeted marketing campaigns, and ultimately, a better bottom line.
Key Aspects of CF Data
Understanding the fundamental concepts of CF data is crucial for effectively implementing it within your own applications. This includes:
- User-Item Interactions: CF data relies heavily on the interactions between users and items. These interactions can range from purchases to ratings, clicks, or even simple views.
- Data Preparation and Cleaning: Raw CF data often requires meticulous cleaning and preparation before being analyzed. Missing values, inconsistencies, and errors can significantly impact the accuracy of the model.
- Algorithm Selection: Different algorithms offer varying levels of accuracy and efficiency. Choosing the right algorithm for your specific needs is vital for optimal results.
- Evaluation Metrics: Assessing the performance of your CF model is paramount. Common metrics include precision, recall, and F1-score, offering insights into the model’s accuracy and effectiveness.
Practical Applications of CF Data
The impact of CF data extends far beyond recommendation engines. Consider these examples:
- E-commerce: Personalized product recommendations drastically increase sales.
- Streaming Services: Content suggestions tailor user experience.
- Social Media: Algorithms suggest relevant content to users.
- Healthcare: Predictive models help in identifying potential health risks.
Conclusion: The Future of CF Data
CF data is evolving rapidly, with ongoing research focusing on improving efficiency, accuracy, and scalability. As technology advances, we can anticipate even more innovative applications of CF data, transforming how we interact with information and services. Unlock the full potential of your data today!